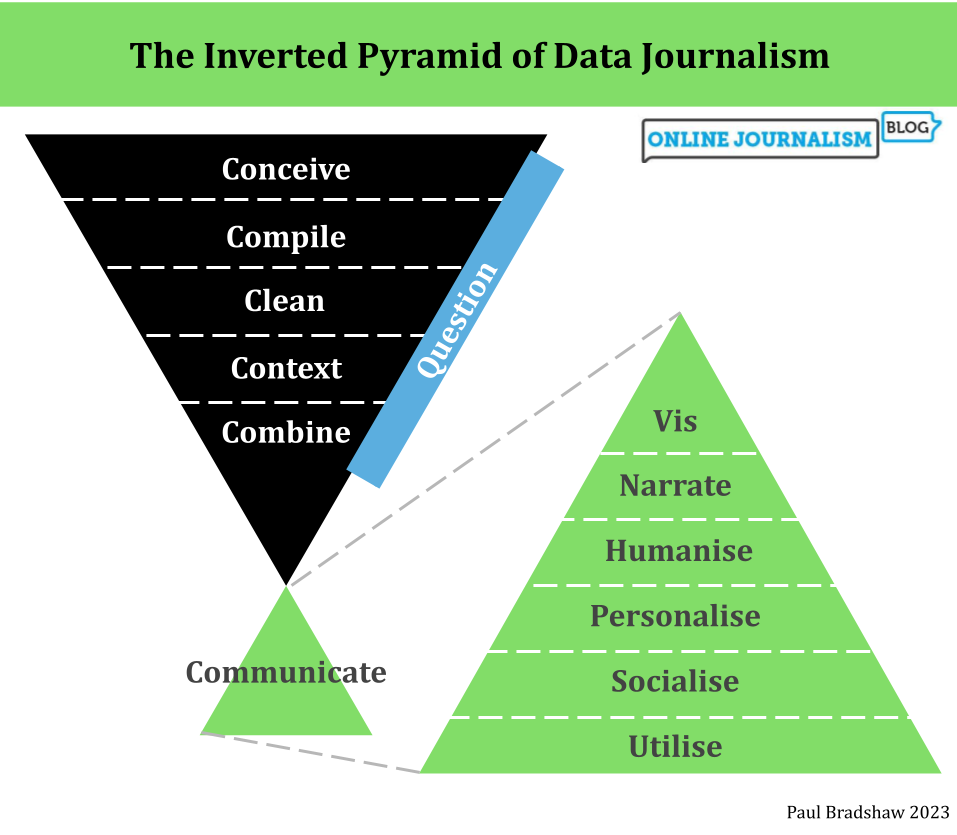
It’s over a decade since I published the Inverted Pyramid of Data Journalism. The model has been translated into multiple languages, taught all over the world, and included in a number of books and research papers. But in that time the model has also developed and changed through discussion and teaching, so here’s a round up of everything I’ve written or recommended on the different stages — along with a revised model in English (shown above; versions have been published before in German, Russian and Ukrainian!).
The most basic change to the Inverted Pyramid of Data Journalism is the recognition of a stage that precedes all others — idea generation — labelled ‘Conceive’ in the diagram above.
This is often a major stumbling block to people starting out with data journalism, and I’ve written a lot about it in recent years (see below for a full list).
The second major change is to make questioning more explicit as a process that (should) take place through all stages — not just in data analysis but in the way we question our sources, our ideas, and the reliability of the data itself.
Alongside the updated pyramid I’ve been using for the past few years I also wanted to round up links to a number of resources that relate to each stage. Here they are…
Continue reading